The MCA framework has been applied to transient Type Ia supernovae detection in the deferred photometric pipeline of the SuperNova Legacy Survey (SNLS) in Moller et al. (2015).
SNLS deferred photometric detection pipeline
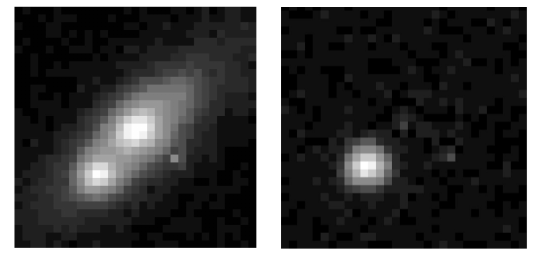
Supernovae are not only extremely luminous objects but they are also transient events, which makes their detection possible by looking for brightness variations on the sky.
The strategy adopted in the SNLS deferred photometric pipeline is to build a high quality reference image of the sky which is then substracted to subsequent exposures. Fig 1. illustrates the substraction of the reference frame for a galaxy hosting a supernova. The image of the galaxy, which remains constant, is substracted out and only the transient supernovae remains after substraction.
The example in Fig. 1 is of course an illustration of a very clean, high SNR, supernova event. In practice however, faint supernovae can not be detected from individual exposures as their SNR is too low. Therefore, in order to increase the signal to noise, the approach implemented in this SNLS pipeline is to build image stacks of a large number of exposures (after reference frame substraction) and to detect supernovae candidates from these stacks.
However, image stacks produced by this SNLS pipeline are plagued by a large number of defects, accumulated at the different levels of the production of stack images (removal of bright stars, resampling defects, imperfect subtractions,…). Some of these defects are illustrated on Figure 2.
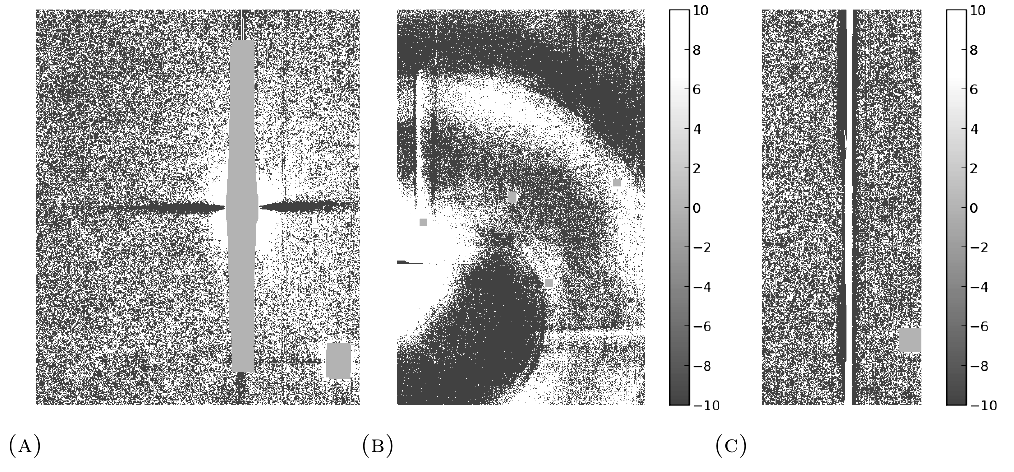
Because of these defects, the detection of supernovae candidates on these images leads to an extremely large number of spurious detections. Figure 3 shows individual detections on these image stacks for one field of SNLS3. For this field, the detection pipeline produced nearly 100,000 detections, 99.6 % of which are purely spurious detections. As can be seen on this figure, spurious detections concentrate around bright stars and detector borders and defects.
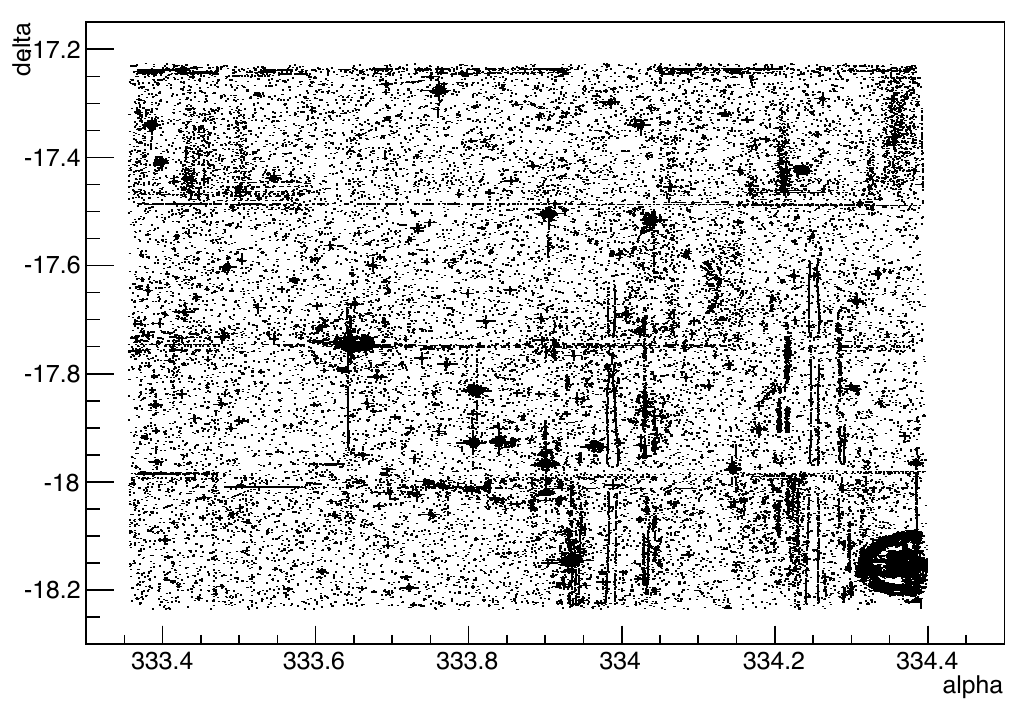
Image cleaning using MCA
In order to reduce the large number of false detections due to defects in the stack images, the approach proposed in Moller et al. (2015) was to clean these images using Morphological Component Analysis prior to performing the detection. to separate supernovae-like signal from defects. Indeed, most defects have morphologies very distinct from that of typical supernovae.
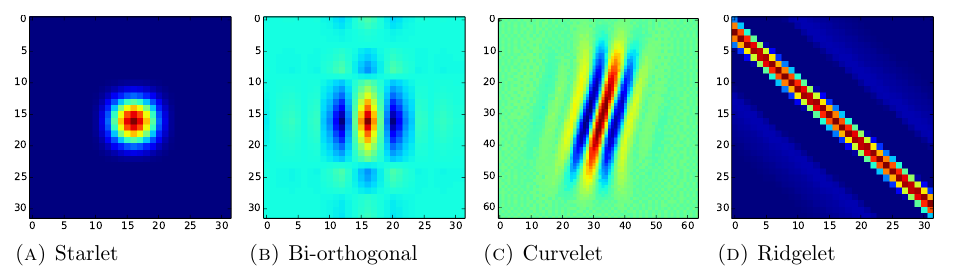
Supernovae signal is very well represented using isotropic wavelets (Starlets) while most of the defects in the image (lines, stars, incorrect substractions) can be extracted using a combination of ridgelets, curvelets and bi-orthogonal wavelets.
In the proposed detection procedure, image stacks are first processed using MCA to extract 4 different morphological components. Then the starlet component, corresponding to supernovae signal, is processed in a similar fashion as in the original pipeline to detect candidates, without being contaminated by the defects removed by the MCA. Figure 5 shows and example of an image stack and the corresponding detection map before cleaning. A clear excess of detections can be found at the vicinity of the bright star in this field. Figure 6 shows the result of the MCA cleaning on the same image, keeping only noise and starlet components. The defects are completely removed and the corresponding detection map is much cleaner.
Results
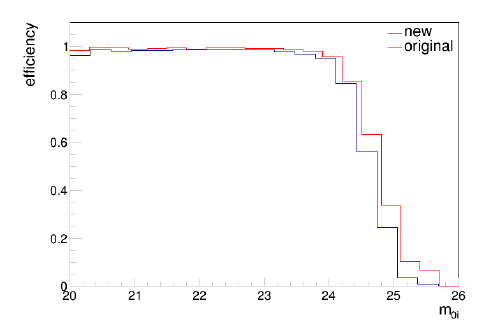
The new detection procedure has been extensively tested using Monte-Carlo simulations. The overall false detection rate is reduced by more than a factor 2 while conserving very similar detection efficiency (see Figure 7, the efficiency is only reduced for very faint SNae which are not suitable for cosmology in any case). A similar improvement could not have been obtained with the original detection pipeline which had already been extensively optimised.
Additionnally, the new detection procedure was found to reduce the magnitude bias of the detected SNe Ia which will have an impact on the cosmological analysis done with this sample.
Publication
- Möller, A., Ruhlmann-Kleider, V., Lanusse, F., Neveu, J., Palanque-Delabrouille, N., Starck, J.-L., 2015, SNIa detection in the SNLS photometric analysis using Morphological Component Analysis. Accepted in JCAP, arxiv:1501.02110